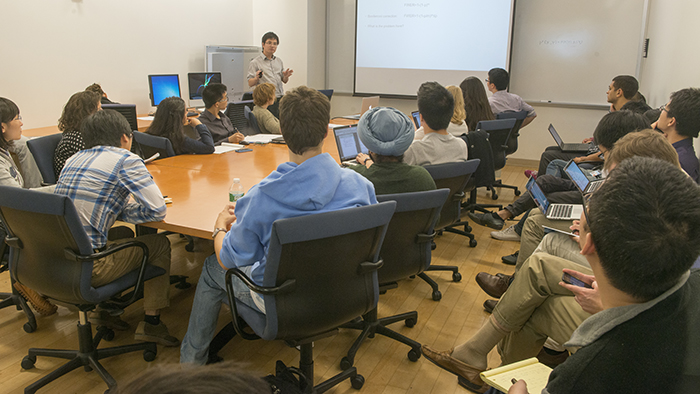
A new team-taught course covers both the experimental and analytical basics of next-generation sequencing. Assistant Professor Chaolin Zhang led the discussion in a recent class. Photo: Lynn Saville.
As the cost of next-generation sequencing has fallen, it has become a ubiquitous and indispensable tool for research across the biomedical sciences. DNA and RNA sequencing — along with other technologies for profiling phenomena such as de novo mutations, protein-nucleic acid interactions, chromatin accessibility, ribosome activity, and microRNA abundance — now make it possible to observe multiple layers of cellular function on a genome-wide scale.
Regardless of a biologist’s chosen area of investigation, such methods have made it possible to explore many exciting new kinds of problems. At the same time, however, it has also dramatically transformed the expertise that young scientists need to develop in order to participate in cutting-edge biological research. Bringing students up to speed with the pace of change in next-generation sequencing has posed a particular challenge for educators.
Now, a new multidisciplinary, graduate-level course organized by the Columbia University Department of Systems Biology is enabling young investigators to begin incorporating these powerful new tools into their studies and future research. Designed by assistant professors Yufeng Shen, Peter Sims, and Chaolin Zhang, the course covers both the experimental principles of next-generation sequencing and key statistical methods for analyzing the enormous datasets that such technologies produce. In this way, it gives students a strong grounding in principles that are critical for more advanced graduate courses as well as the ability to begin applying deep sequencing technologies to investigate the questions they are interested in pursuing.
As Dr. Sims explains, “Whether you are a graduate student in systems biology, biochemistry, or microbiology, the chance that you are going to be doing next-generation sequencing is pretty high. At the same time, it’s completely not taught at the undergraduate level. There is no text book nor is there any time in a typical undergraduate biology curriculum to get into this in any kind of detail. Even at top-tier universities students come into graduate school without having any experience with it, and often they’re expected to jump right into this kind of research. We decided that this was a problem we had to fix.”