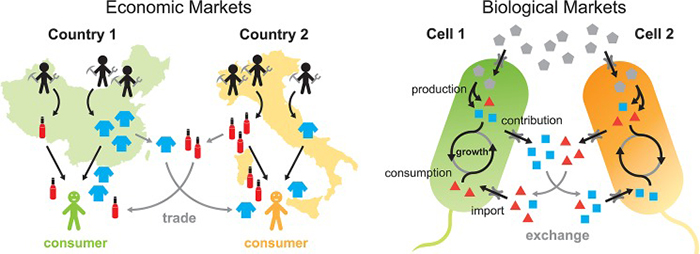
In a similar manner to the ways in which countries make and trade goods, microbial cells within bacterial communities exchange metabolites to promote cell growth. This perspective could provide a way of studying microbial communities from the perspective of economics.
An article in the Wall Street Journal reports on a recent collaboration involving Columbia University Department of Systems Biology Assistant Professor Harris Wang and Claremont Graduate University economist Joshua Tasoff that identified some intriguing similarities between economic markets and the exchange of resources among microbes within bacterial communities.
In an unusual marriage, biology and economics appear to be a match made in heaven.
Four years ago, two former roommates reunited at a friend’s wedding had time to catch up. The first, an economist, asked: “What are you working on?” The second, a biologist, answered: “How microbial communities interact. It’s kind of like in economics.”
And that’s when the intellectual sparks began to fly.
Turns out microbial communities—what most of us think of as germs—expand by trading metabolites such as amino acids with other species of bacteria, just like free-market economies grow by exchanging goods and services.
That novel insight, inspired by a chance conversation and supported with research developed over the intervening years, provides a framework to explain how different species of bacteria interact in complex communities.
You can read the entire article here: Economies of Ail: How Bacteria Flourish. [login may be required]
Related publication
Tasoff J, Mee MT, Wang HH. An economic framework of microbial trade. PLoS One. 2015 Jul 29;10(7):e0132907.