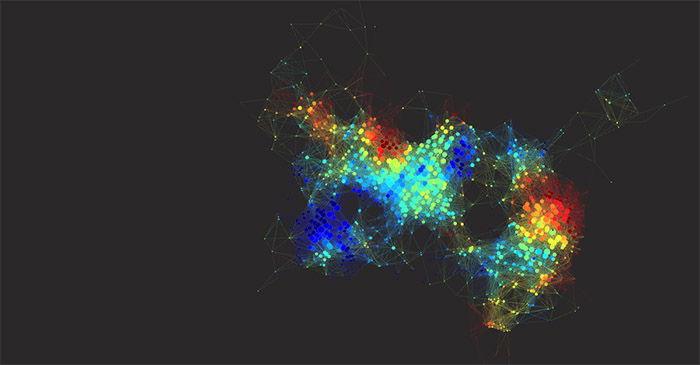
Shown here, topology data analysis of cancer samples; Image credit: The Rabadan Lab
The new Program for Mathematical Genomics (PMG) is aiming to address a growing—and much-needed—area of research. Launched in the fall of 2017 by Raul Rabadan , a theoretical physicist in the Department of Systems Biology, the new program will serve as a research hub at Columbia University where computer scientists, mathematicians, evolutionary biologists and physicists can come together to uncover new quantitative techniques to tackle fundamental biomedical problems.
"Genomic approaches are changing our understanding of many biological processes, including many diseases, such as cancer," said Dr. Rabadan, professor of systems biology and of biomedical informatics. "To uncover the complexity behind genomic data, we need quantitative approaches, including data science techniques, mathematical modeling, statistical techniques, among many others, that can extract meaningful information in a systematic way from large-scale biological systems."
This new program is being built upon collaborative research opportunities to explore and develop mathematical techniques for biomedical research, leading to a deeper understanding of areas such as disease evolution, drug resistance and innovative therapies. Inaugural members of the new program include faculty across several disciplines: statistics, computer science, engineering and pathology, to name a few. The program also will provide education and outreach to support and promote members' work, including joint discussion groups, the development of cross-campus courses and scientific meetings.
In honor of its launch, PMG will co-host a two-day symposium February 7 to 8 on cancer genomics and mathematical data analysis. Guest speakers from Columbia University, Memorial Sloan Kettering and Cornell University will present a comprehensive overview of quantitative methods for the study of cancer through genomic approaches.