Congenital diaphragmatic hernia (CDH) is a severe birth defect. For babies born with CDH, their diaphragms are not developed properly, with some or all parts of the abdominal organs pushed into the chest. The displacement of these critical organs can have a significant impact on how the lungs develop and grow.
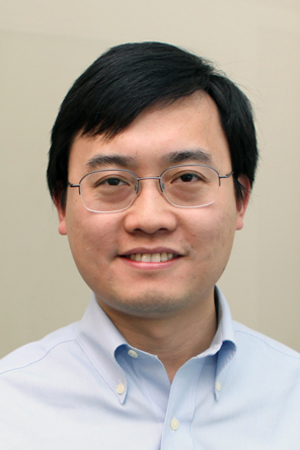
Yufeng Shen, PhD, associate professor of systems biology
A recent study , led by Yufeng Shen , PhD, and Wendy Chung , MD, PhD, and their labs at Columbia University Irving Medical Center , investigated the genetic risk factors linked to CDH and analyzed data from whole genome sequencing and exome sequencing to determine novel mutations. The study also uncovered the link between CDH and additional developmental disorders.
“Many babies with this birth defect also have lung hypoplasia or pulmonary hypertension and babies have difficulty breathing. Even with advanced care available, the mortality rate is still about 20 percent,” says Dr. Shen, associate professor of systems biology at Columbia, with a joint appointment in the Department of Biomedical Informatics .
“One hypothesis is that the lung condition is not necessarily caused by the physical compression on the developing lungs in the chest,” explains Dr. Shen, “it can be caused by the same genetic defect that causes CDH. Finding those genes is absolutely necessary to improve care and develop effective treatment in the long run.”
Scientists have been aiming to identify new risk genes in CDH—and other developmental disorders—with the hope that with improved genetic diagnosis more tailored or long-term care for patients born with this defect could be provided, as well as potential targets for intervention down the road.